Introduction: characterization of single cells
Phenotypic variation exists even in clonal cell populations that are derived from a single ancestral cell [1]. Such variation is interesting from a medical perspective as it can create subsets of the population that behave differently compared to others (e.g. some cells may develop resistance to drugs [2]). Quantifying dynamic changes in variation and thus, cell fate, requires individual cells to be tracked at high spatial and temporal resolution.
Some live cell imaging techniques (e.g. high content imaging) do allow to track individual cells through time, but they also require cells to be fluorescently-labelled, which generates phototoxicity, imposing limitations on either the duration of the experiment, or the frequency of imaging during an experiment.
Nanolive imaging is not fluorescence-based, which avoids these limitations. Our microscopes measure the cells refractive index [3], an intrinsic material property of the cell. This permits to monitor cells at high frequency for unlimited periods of time, without compromising spatial resolution. This is advantageous as it allows to follow the state, behaviour, phenotype and function of single cells at the population level.
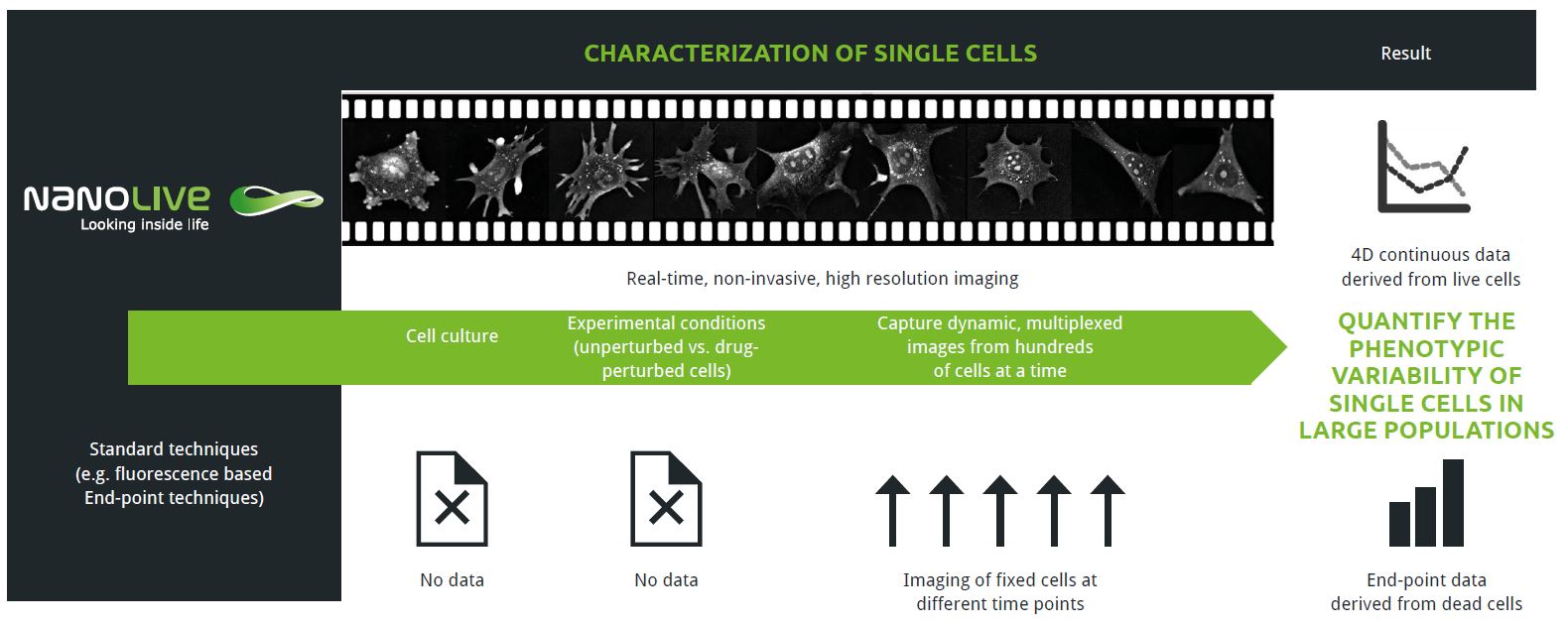
In the Feature Application entitled Characterizing Single Cells, we showcase the enormous potential that Nanolive live cell imaging holds for single cell characterization. In the case study we present today, we we extend our analysis to include a quantitative assessment of lipid droplet dynamics. The following case studies can be accessed downloading the application note document here.
We hope this application note will inspire researchers to revolutionize their research using Nanolive cell imaging and improve single cell analyses by capturing the full complexity of time-varying cell states and the interconnectedness of cell subsets within a population context.
Background: lipid droplet dynamics
Understanding lipid droplet (LD) dynamics is of great interest for the health (e.g., diabetes and obesity), food (e.g., vegetable oil), cosmetics (e.g., soap) and green chemistry (e.g., biodiesel) industries [4]. Fluorescence-based microscopy has long been the conventional way of studying LDs but suffers from phototoxicity and photobleaching limits [5]. Coherent Anti-Stokes Raman Scattering (CARS) microscopy offers a label-free alternative, but it is complicated, expensive, and signal specificity is a concern [6]. Nanolive label-free imaging overcomes all these drawbacks, and is fully quantitative, ensuring no detail is missed at the population, single cell, and individual LD level.
1. Quantifying LD dynamics at the population level
An unperturbed population of 3T3-derived pre-adipocyte cells were imaged for 30 h using the 10×10 grid-scan mode on Nanolive’s CX-A (one image taken every 8.5 mins). Resulting images were subjected to a maximum intensity Z-projection, segmented into individual cells and LDs and analyzed using tools developed in house (Video 1, Image 1).
Video 1
Quantifying LD dynamics at the population level. Unperturbed population of 3T3-derived pre-adipocyte cells were imaged for 30 h (one image taken every 8.5 mins). Detail of segmented lipid droplets. Graphs: total LD count over time and total LD dry mass over time.
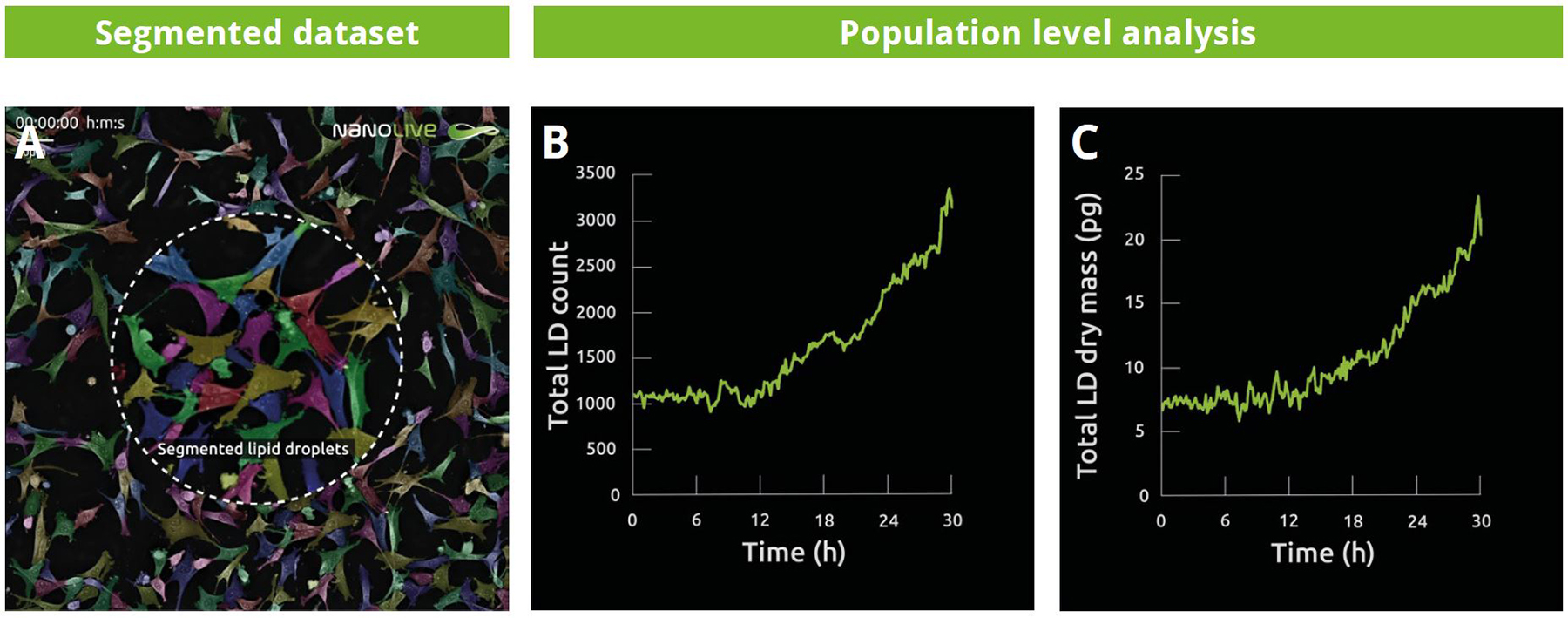
Image 1
Unperturbed population of 3T3-derived pre-adipocyte cells were imaged for 30 h (one image taken every 8.5 mins). Detail of segmented lipid droplets. Graphs: total LD count over time and total LD dry mass over time.
Observations
- LD segmentation is highly precise (A), irrespective of cell confluence, which increased by 26.7% (from 278 to 518 cells), over the course of the experiment (data not shown).
- The graphs on the right show a basic, population-level analysis of total LD count (B) and total LD dry mass content (C). Both metrics are relatively stable over the first 12 h of the experiment and increase over the next 24 h, a pattern that mimics the change in cell confluence.
- The magnitude of the increase of the two traits is similar. The total number of LDs increases by 187% (B), while the total LD dry mass increases by 202% over the same period (C).
2. Quantifying LD dynamics at the single cell level
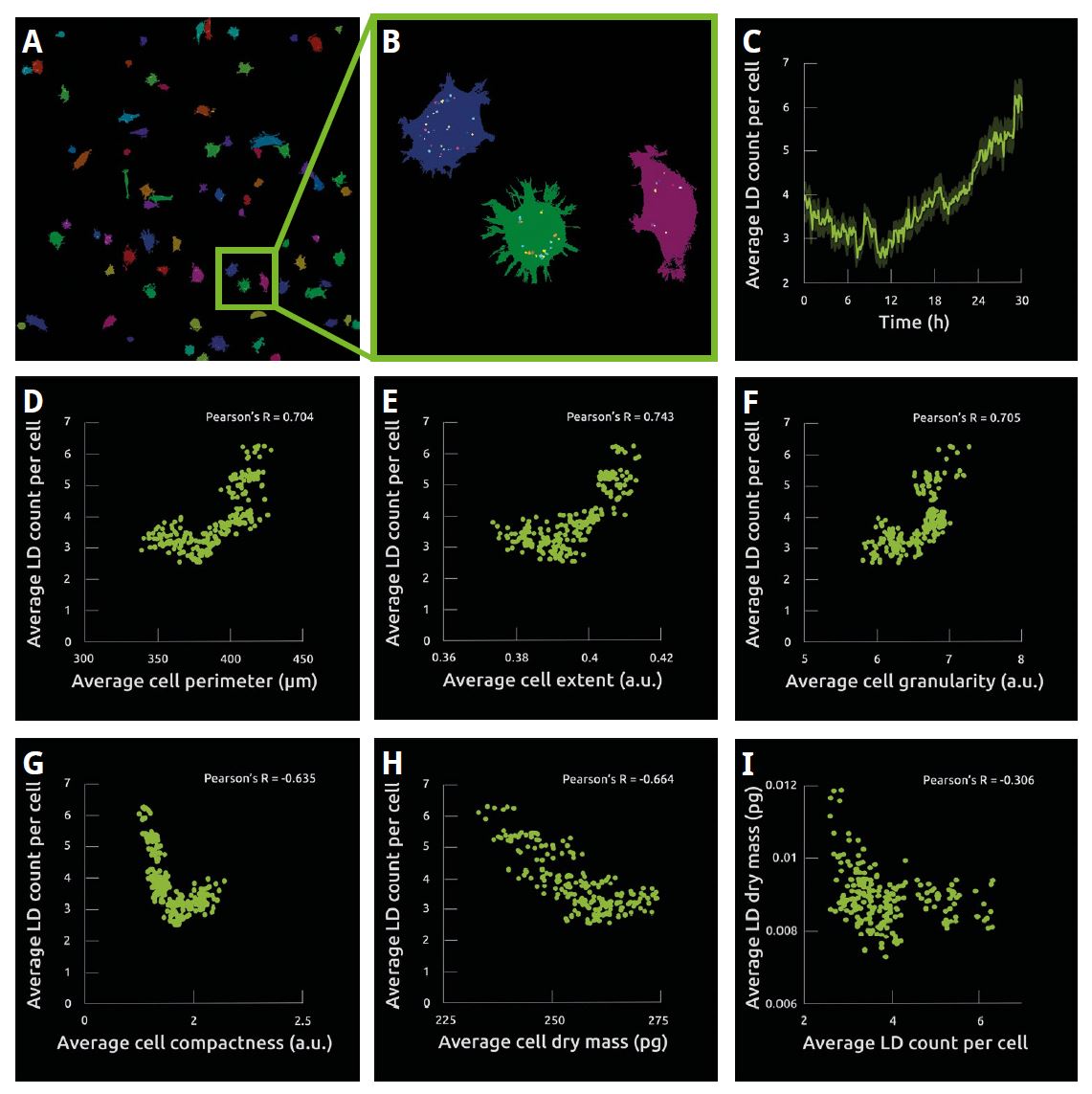
Image 2
Quantifying LD dynamics at the single cell level. Graphs: C) average LD count per cell over time, D) correlation between average LD count per cell and average cell perimeter, E) correlation between average LD count per cell and average cell extent, F) correlation between average LD count per cell and average cell granularity, G) correlation between average LD count per cell and average cell compactness, H) correlation between average LD count per cell and average cell dry mass and I) correlation between average LD dry mass and average LD count per cell.
Observations
- Taking the analysis one step further from the population level (A) to the single cell level (B) reveals there is an inital reduction in the average number of LDs per cell over the first 12 h and subsequent increase over the rest of the experiment (C) (for details, see Image 2 and Video 2). This increase could be linked to cell senescence; several studies have reported LD accumulation in older cells [7,8]. Alternatively, this could reflect a change in energy homeostasis [9].
- Correlating the average number of LDs with other cell metrics, reveals that cells containing more LDs tend to have a larger perimeter (D), a greater extent (E), and higher granularity (F), but are less compact (G), and contain less dry mass (H). This last finding, concerning dry mass, is particularly interesting. There are two possible explanations. Either individual LDs are smaller in cells that contain more, or cells containing less LDs have more cytosolic and nuclear dry mass.
Video 2
Quantifying LD dynamics at the single cell level. Graphs: average LD count per cell over time, correlation between average LD count per cell and average cell perimeter, correlation between average LD count per cell and average cell extent, correlation between average LD count per cell and average cell granularity, correlation between average LD count per cell and average cell compactness, correlation between average LD count per cell and average cell dry mass and correlation between average LD dry mass and average LD count per cell.
3. Quantifying LD dynamics at the individual LD level
The data can also be used to examine the response of large populations of cells over time (see Image 3 and Video 3). The graphs in Image 3 show cell extent and compactness over time, first as line graphs showing the mean ± standard error (SE) and then as the co-efficient of variation (CV).
Observations
- The most detailed analysis we can do, is to describe the morphology/aspects of individual LDs (A; white arrows). Here, we calculate how the average dry mass content of a LD changes over time. In this example, we see that dry mass content fluctuates, but does not change drastically over the course of the experiment (B).
- Plotting individual LD dry mass content against the average LD count per cell reveals that individual LDs tend to be smaller if a cell contains more of them.
Video 3
Quantifying LD dynamics at the individual LD level. Graphs: average LD dry mass over time and correlation between average LD dry mass and average LD count per cell
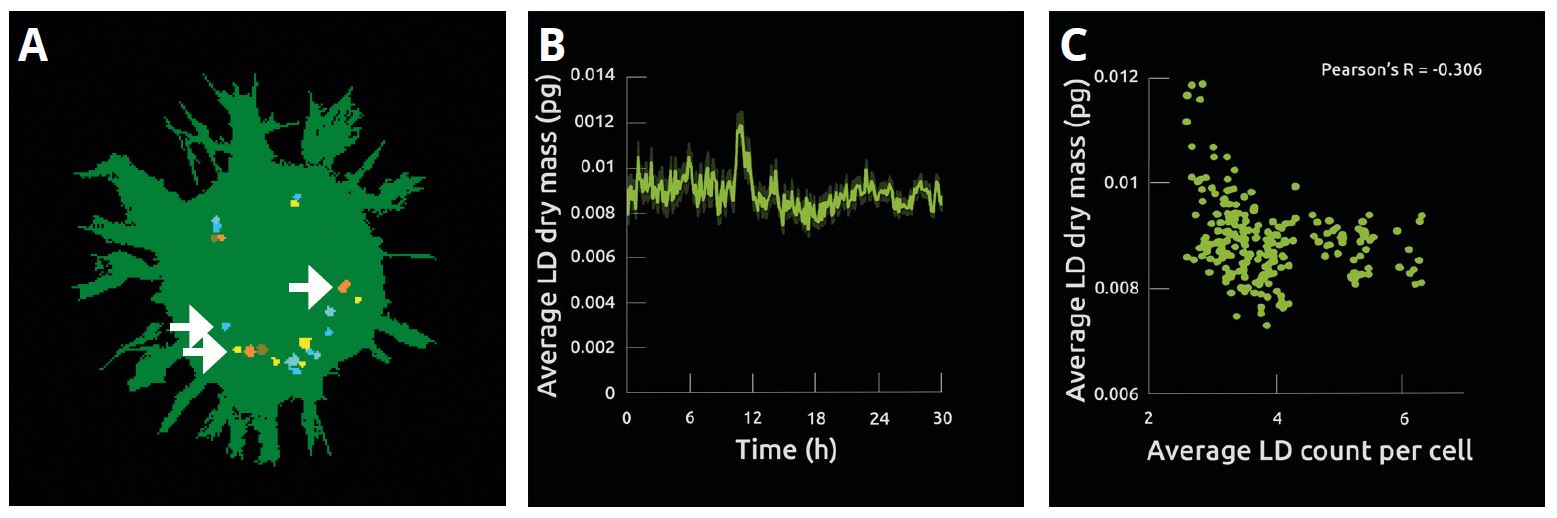
Image 3
Quantifying LD dynamics at the individual LD level. Graphs: average LD dry mass over time and correlation between average LD dry mass and average LD count per cell
Conclusions
- Provides quantitative morphological and biochemical information (e.g. count per cell and dry mass content) about individual LDs, in vitro.
- LD metrics can be combined/correlated with other cell morphological metrics (e.g. cell count, confluence, area, perimeter, extent, compactness, dry mass, and granularity), which are captured simultaneously.
- This technique permits to investigate the role of lipid metabolism in health and diseases, and to test the effects of different oil compositions on LD formation [4].
References
[1] Geiler-Samerotte KA et al. Curr. Opin. Biotech. 24(4):752-9 (2013)
[2] Bishop AL et al. Mol. Microbiol. 63, 507-520 (2007)
[3] Cotte Y et al. Nat. Photonics. 7(2):113-7 (2013)
[4] Gurr, M.I. et al. 2016. Lipids: biochemistry, biotechnology and health. John Wiley & Sons.
[5] Laissue PP. et al. 2017. Nat. Meth. 14(7):657-61.
[6] Folick A. et al. 2011. Curr. Opin. Gen. Develop. 1;21(5):585-90.
[7] Flor, AC. et al. 2017. Cell Death Discov. 30;3(1):1-2.
[8] Lizardo, DY. et al. 2017. Mol. BioSyst. 13:498–509.
[9] Olzmann, JA. and Carvalho, P. 2019. Nat. Rev. Mol. Cell Biol. 20(3):137-55.
Read our latest news
Revolutionizing lipid droplet analysis: insights from Nanolive’s Smart Lipid Droplet Assay Application Note
Introducing the Smart Lipid Droplet Assay: A breakthrough in label-free lipid droplet analysis Discover the power of Nanolive's Smart Lipid Droplet Assay (SLDA), the first smart digital assay to provide a push-button solution for analyzing lipid droplet dynamics,...
Food additives and gut health: new research from the University of Sydney
The team of Professor Wojciech Chrzanowski in the Sydney Pharmacy School at the University of Sydney have published their findings on the toxic effect of titanium nanoparticles found in food. The paper “Impact of nano-titanium dioxide extracted from food products on...
2023 scientific publications roundup
2023 has been a record year for clients using the Nanolive system in their scientific publications. The number of peer-reviewed publications has continued to increase, and there has been a real growth in groups publishing pre-prints to give a preview of their work....
Nanolive microscopes
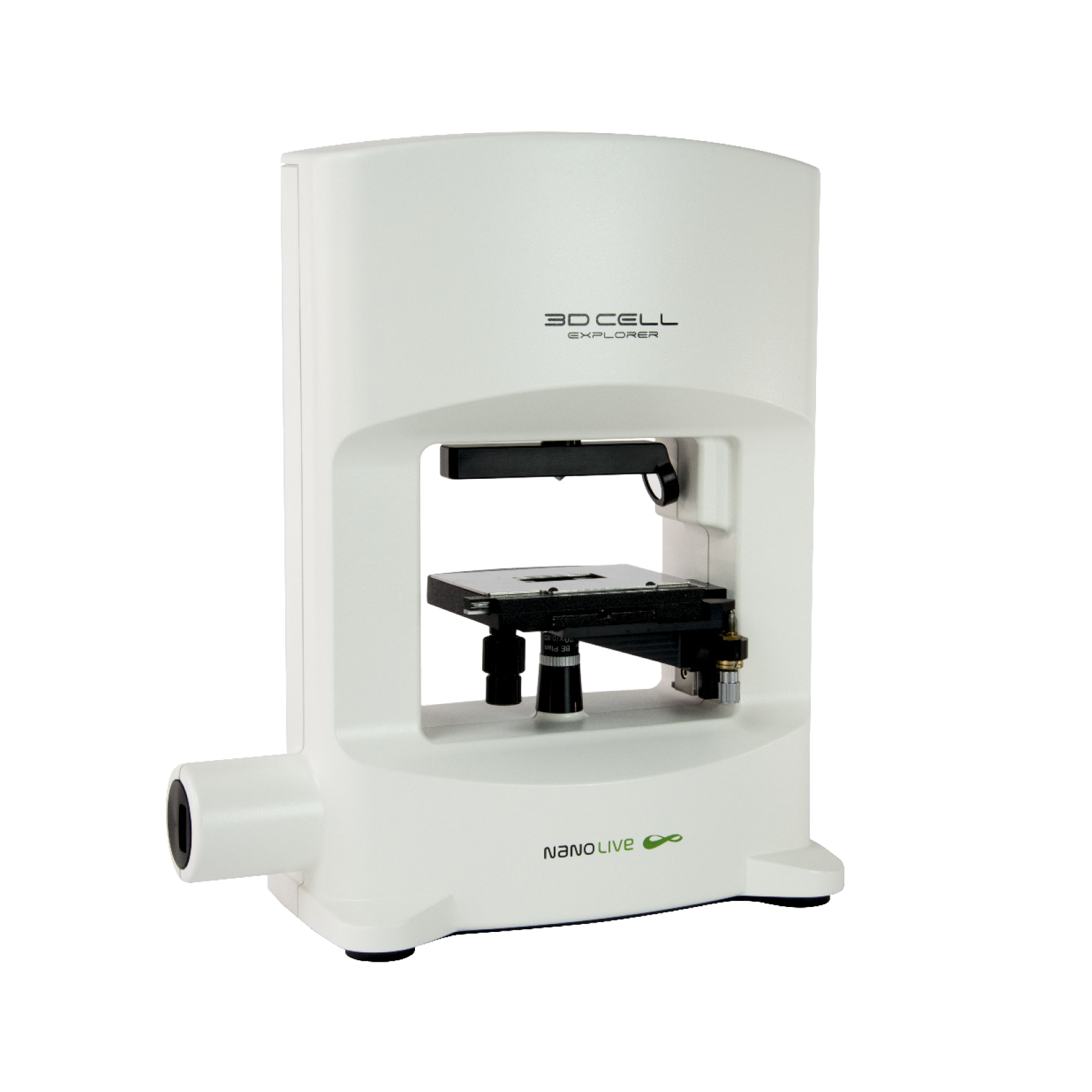
3D CELL EXPLORER
Budget-friendly, easy-to-use, compact solution for high quality non-invasive 4D live cell imaging
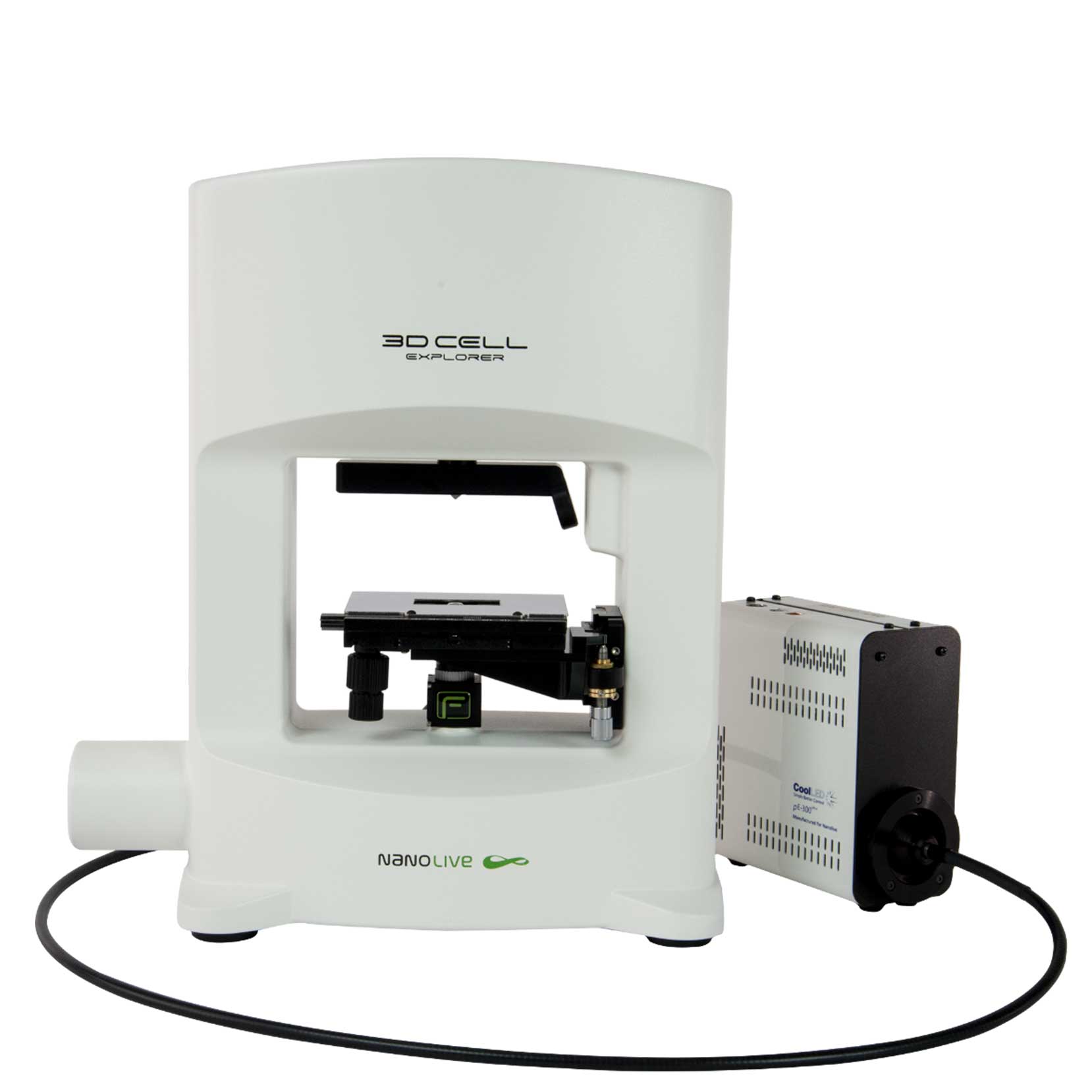
3D CELL EXPLORER-fluo
Multimodal Complete Solution: combine high quality non-invasive 4D live cell imaging with fluorescence
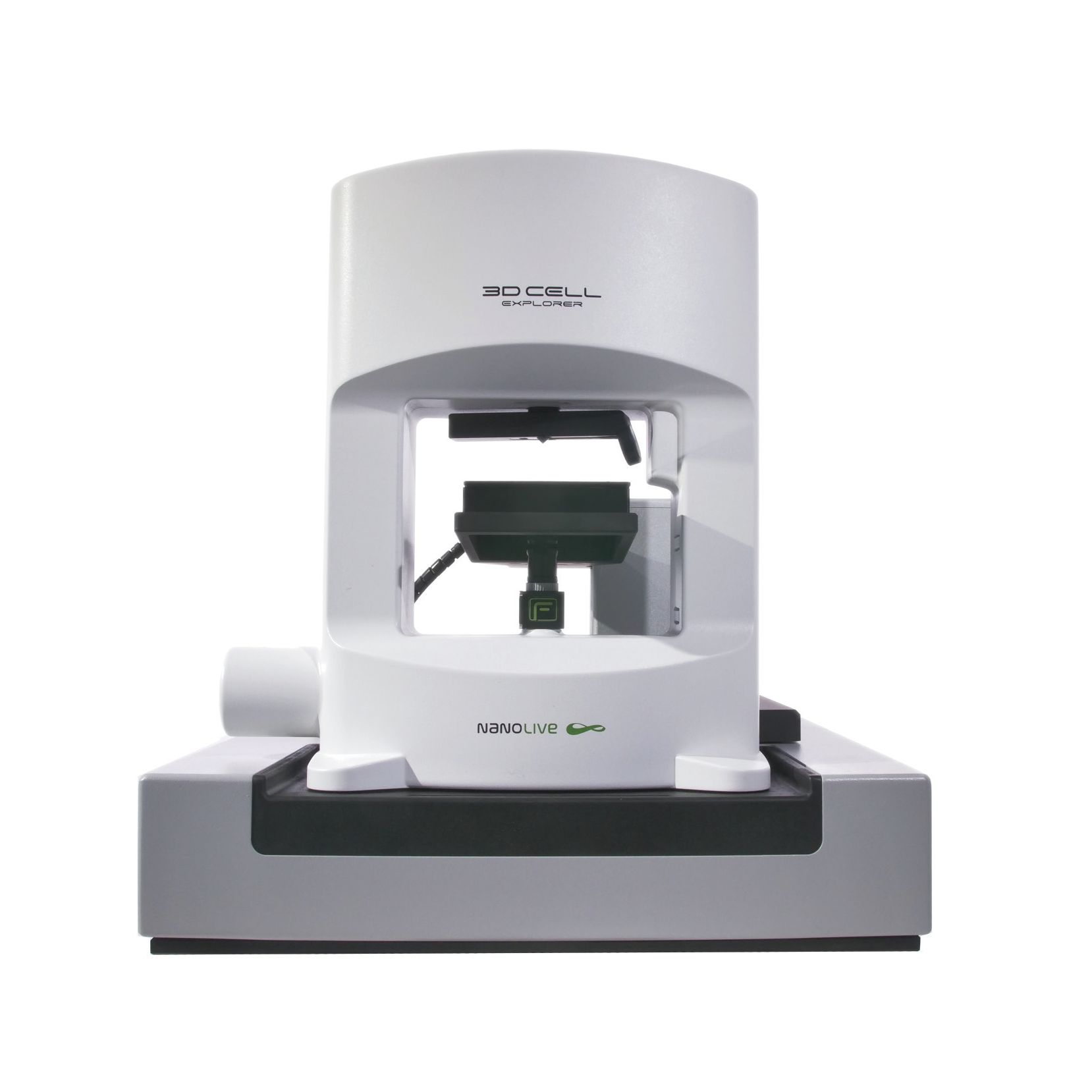
CX-A
Automated live cell imaging: a unique walk-away solution for long-term live cell imaging of single cells and cell populations